![]() |
Cherith Professor and Founding Chair, Department of Biomedical Informatics
Vice Chair for Laboratory Initiatives and Digital Medicine in the Department of Pathology Vice President for Clinical Informatics, Stony Brook Medicine Associate Director, Stony Brook Cancer Center Department of Biomedical Informatics
HSC L3-043
Stony Brook, NY 11794
Phone: (631) 638-1420
Website (URL): https://bmi.stonybrookmedicine.edu/Joel_Saltz_Research Email: joel.saltz@stonybrookmedicine.edu |
Dr. Saltz is a pioneer in developing Digital Pathology tools, methods and algorithms with the ultimate goal of extracting and leveraging digitalized Pathology information to better predict cancer outcome and to steer cancer therapy. He is also an expert in high end computing and has developed a variety of highly cited systems software methods.
His research in Pathology spans twenty years and consists of closely coordinated efforts in image analysis, machine learning, database design and high end computing. He has developed tools and methods through years of funded projects supported by a wide range of institutes and agencies including NCI, NLM, NIBIB, NSF, DARPA, AFOSR, NASA, DOD and DOE. His seminal work in digital imaging laid the foundation for digital pathology as it is today. He was the first to develop the “Virtual Microscope,” and pioneered developments in digital pathology whole slide image navigation, data management and computer aided classification.
Dr. Saltz’s initial efforts included development of the first whole slide image viewer, and devising efficient methods for management, caching and supporting analytics carried out on whole slide datasets. This work became the foundation of the new field of Pathology Imaging Informatics, today investigators he mentored can be found carrying out exciting research in institutions across the country.
Over the years, Dr. Saltz has developed a rich set of Pathology informatics tools, methods and algorithms. For instance, he and his group have recently developed a rich set of pipelines to compute a variety of biologically significant Pathology features, including spatial maps of tumor infiltrating lymphocytes (TILs). The quantification of TILs is well documented to have prognostic value in many contexts; understanding patient immune response to tumors is increasingly important with the advance of cancer immunotherapy. For most cancer types, Pathologists do not comment on TILs in their reports and even in cancer types where TIL comments are sometimes made, they only use high level terms such as “brisk” or “sparse”. Dr. Saltz is able to precisely quantify percent tumor infiltrating lymphocytes and is also able to generate detailed TIL Maps. He has used these methods to generate publicly available whole slide TIL maps and spatial statistics for 13 cancer types and 5,000 subjects – these are available on The Cancer Imaging Archive. He is now extending and leveraging these methods in many other studies, as well as for the NCI SEER program.
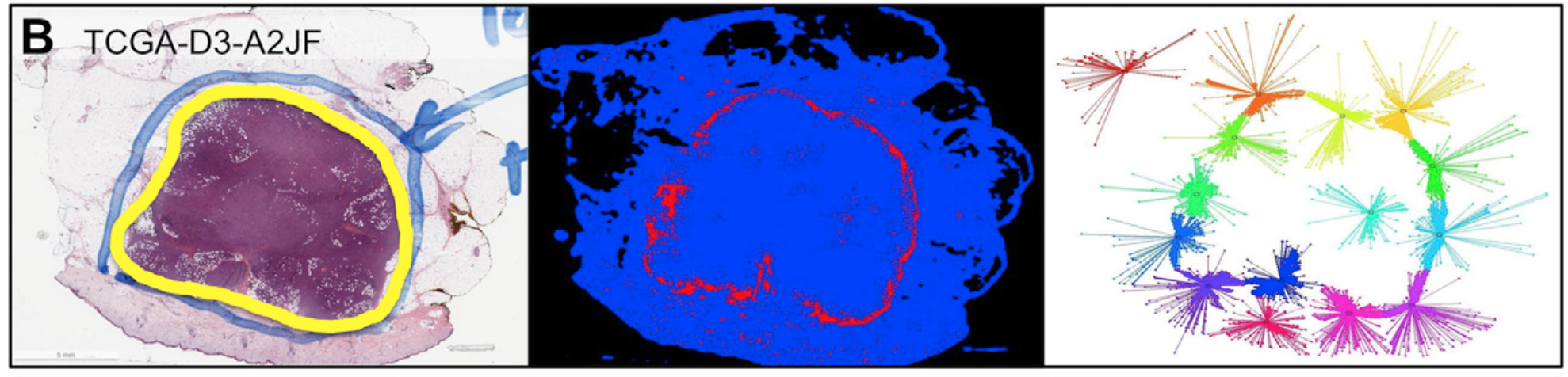
Dr. Saltz and collaborators have also developed a variety of machine learning methods to target analysis of whole slide images. This includes a variety of methods that target Neuroblastoma classification as well as methods that are able to leverage coarse grained whole slide training data through combining patch-level convolutional neural networks with supervised decision fusion. He achieved state-of-the-art results in predicting the subtypes of brain and lung tumors. Dr. Saltz has also developed innovative computational methods for carrying out data and computationally challenging image analysis and machine learning methods on whole slide Pathology images. These methods are capable of taking advantage of highly scalable hybrid CPU/GPU architectures and are able to leverage capabilities provided by cloud and leadership class architectures including the Oak Ridge National Laboratory Summit platform.
Finally, Dr. Saltz made foundational contributions to data science through development of innovative methods for moving computation to data, development of a very early prototype map/reduce type framework, foundational methods for runtime compiler analysis of adaptive applications and analyses of key data structures employed in the management of spatial data (Hilbert Space-Filling Curves).
Joel Saltz received his MD and Computer Science PhD from the Medical Scientist Training Program at Duke University. He was trained in Clinical Pathology at Johns Hopkins Medical School and served on the Johns Hopkins faculty as Professor and Director of Pathology Informatics. He has launched new Departments of Biomedical Informatics at Stony Brook, Emory and Ohio State and has also served on the Computer Science faculty at Yale University and the University of Maryland College Park.
Joel Saltz, Dimitris Samaras, Tahsin Kurc, Chao Chen and Fusheng Wang form the Digital Pathology group at Stony Brook. This closely integrated research group targets development of AI and machine learning algorithms, numerical methods, software tools and database architectures for digital Pathology and multi-scale tissue analysis.
1. Kapse, S., Das, S., Zhang, J., Gupta, R. R., Saltz, J., Samaras, D., & Prasanna, P. Attention de-sparsification matters: Inducing diversity in digital pathology representation learning. Medical Image Analysis, 93, 103070. doi:10.1016/j.media.2023.103070 (2024). PMID: 38176354
2. Kaczmarzyk, J. R., O’Callaghan, A., Inglis, F., Gat, S., Kurc, T., Gupta, R., Bremer, E., Bankhead, P., & Saltz, J. H. Open and reusable deep learning for pathology with WSINFER and QuPath. Npj Precision Oncology, 8(1), doi:10.1038/s41698-024-00499-9 (2024). PMID: 38200147 PMCID: PMC10781748
3. Yellapragada, S., Graikos, A., Prasanna, P., Kurc, T., Saltz, J., & Samaras, D. PATHLDM: Text conditioned latent diffusion model for histopathology. doi:10.48550/arXiv.2309.00748 (2023).
4. Abousamra, S., Gupta, R., Kurc, T., Samaras, D., Saltz, J., & Chen, C. Topology-guided multi-class cell context generation for digital pathology. 2023 IEEE/CVF Conference on Computer Vision and Pattern Recognition (CVPR). doi:10.1109/cvpr52729.2023.00324 (2023).
5. Abousamra, S., Belinsky, D., Van Arnam, J., Allard, F., Yee, E., Gupta, R., Kurc, T., Samaras, D., Saltz, J., Chen, C. Multi-class cell detection using spatial context representation. 2021 IEEE/CVF International Conference on Computer Vision (ICCV). doi:10.1109/iccv48922.2021.00397 (2021).
6. Hou, L., Agarwal, A., Samaras, D., Kurc, T. M., Gupta, R. R., & Saltz, J. H. Robust histopathology image analysis: To label or to synthesize? 2019 IEEE/CVF Conference on Computer Vision and Pattern Recognition (CVPR). doi:10.1109/cvpr.2019.00873 (2019). PMID: 34025103 PMCID: PMC8139403
7. Saltz J, Sharma A, Iyer G, Bremer E, Wang F, Jasniewski A, DiPrima T, Almeida JS, Gao Y, Zhao T, Saltz M, Kurc T. A Containerized Software System for Generation, Management and Exploration of Features from Whole Slide Tissue Images. Cancer Research. 2017 Nov 1; 77(21) PMID: 29092946; PMCID: PMC5987533
8. Cooper LA, Kong J, Gutman DA, Wang F, Gao J, Appin C, Cholleti S, Pan T, Sharma A, Scarpace L, Mikkelsen T, Kurc T, Moreno CS, Brat DJ, Saltz JH. Integrated morphologic analysis for the identification and characterization of disease subtypes. Journal of the American Medical Informatics Association: JAMIA 19, 317-323, doi:10.1136/amiajnl-2011-000700 (2012). PMID: 22278382; PMCID:PMC3277636
9. Saltz, J., Gupta, R., Hou, L., Kurc, T., Singh, P., Nguyen, V., Samaras, D., Shroyer, K.R., Zhao, T., Batiste, R. and Van Arnam, J., 2018. Spatial organization and molecular correlation of tumor-infiltrating lymphocytes using deep learning on pathology images. Cell reports, 23(1), p.181. DOI: 10.1016/j.celrep.2018.03.086 PMCID: PMC5943714
10. Hou,L., Samaras, D., Kurc, T.,GaoY., Davis, J., Saltz,J., Patch-based Convolutional Neural Network for Whole Slide Tissue Image Classification. In Proceedings of the IEEE Conference on Computer Vision and Pattern Recognition (CVPR), 2016), Las Vegas, NV
11. Kong, J. et al. Machine-based morphologic analysis of glioblastoma using whole-slide pathology images uncovers clinically relevant molecular correlates. PloS one 8, e81049, doi:10.1371/journal.pone.0081049 (2013).
12. Cooper, L. A. et al. Integrated morphologic analysis for the identification and characterization of disease subtypes. Journal of the American Medical Informatics Association : JAMIA 19, 317-323, doi:10.1136/amiajnl-2011-000700 (2012).
13. Cooper, L. A. et al. The tumor microenvironment strongly impacts master transcriptional regulators and gene expressiocn class of glioblastoma. The American journal of pathology 180, 2108-2119, doi:10.1016/j.ajpath.2012.01.040 (2012).
14. Cooper, L. A. et al. An integrative approach for in silico glioma research. IEEE transactions on bio-medical engineering 57, 2617-2621, doi:10.1109/tbme.2010.2060338 (2010).
15. Moon, B., Jagadish, H. V., Faloutsos, C. & Saltz, J. H. Analysis of the clustering properties of the Hilbert space-filling curve. Knowledge and Data Engineering, IEEE Transactions on 13, 124-141 (2001).
16. Kurc, T., Çatalyürek, Ü., Chang, C., Sussman, A. & Saltz, J. Visualization of large data sets with the active data repository. Computer Graphics and Applications, IEEE 21, 24-33 (2001).
17. Beynon, M. D. et al. Distributed processing of very large datasets with DataCutter. Parallel computing 27, 1457-1478 (2001).
18. Saltz, J. H., Mirchandaney, R. & Crowley, K. Run-time parallelization and scheduling of loops. Computers, IEEE Transactions on 40, 603-612 (1991).
Scholar | NCBI | DBLP | PubMed
Stony Brook Medicine: CS 595 Topics in Computer Science: Data Analytics Software - Stacks
At Emory, Georgia Tech, Ohio State and U Maryland: Graduate seminars in Data Analytics, Graduate seminars in High Performance Computing
Biomedical Informatics I and II, Senior undergraduate database course, Senior undergraduate operating systems, Senior undergraduate computer architectures